This use case presents a comprehensive solution centered on the RZ/V2M evaluation board, equipped for developing cutting-edge Vision AI applications. The RZ/V2M offers features like efficient camera sensor processing, low-power AI inference on its MCU, and real-time video streaming. Furthermore, the integration of the Sony IMX568 global shutter image sensor in the design tackles the challenge of accurate object detection in dynamic environments. This sensor technology excels at capturing clear, distortion-free images even under rapid motion.
To validate the effectiveness LiveBench provides a comprehensive testing environment with features like remote testing, real-time data visualization, and an external image rotation system. This system allows engineers to rigorously assess the under dynamic motion scenarios, replicating real-world situations encountered by autonomous vehicles (AV).
By combining the strengths of the RZ/V2M board, the Sony IMX568 sensor, and the functionalities of LiveBench, this solution empowers the development of next-generation Vision AI applications that unlock the full potential of AVs. This paves the way for a future where AVs operate with greater intelligence, precision, and safety, revolutionizing industries and shaping the way we interact with the world around us.
The Challenge
Vision AI in Autonomous Vehicles for Safety and Efficiency in Navigation
Decades of technological advancements have propelled us from human-driven vehicles to the cusp of fully autonomous cars. The evolution of active and passive safety systems in modern vehicles laid the groundwork for this automation revolution. These intelligent machines are poised to reshape industries, redefine transportation, and revolutionize our daily lives.
Up to 15% of all new vehicles sold in 2030 could be fully autonomous.
McKinsey Report on Automotive revolution – perspective towards 2030
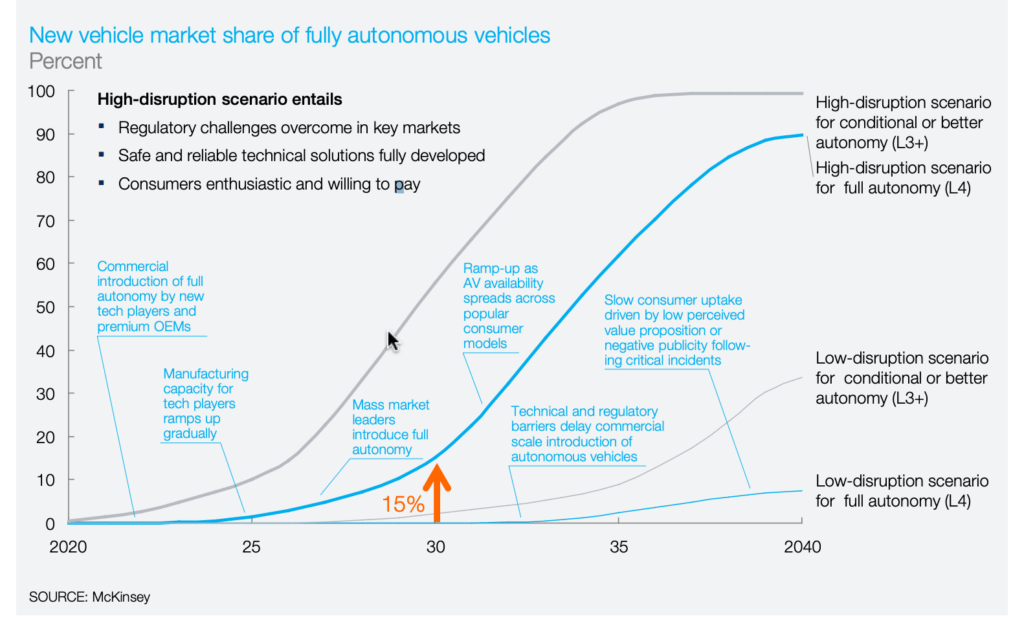
This rapid growth highlights the increasing need for reliable and efficient Vision AI systems in self-driving cars. However, a critical challenge lies at the heart of this advancement: robust and reliable Vision AI.
Over 1.35 million people die annually from road traffic accidents, which is about 3,700 people per day.
– The World Health Organization (WHO)
Vision AI in AVs can reduce these accidents by enabling accurate object detection and real-time decision-making. Vision AI empowers AVs to “see” and understand their surroundings. It enables them to perform real-time object detection, classification, and tracking, all of which are essential for safe and efficient navigation.
Achieving these capabilities requires addressing several key hurdles:
Accurate Object Detection
AVs must accurately identify objects in their environment, regardless of size, shape, or orientation. This is particularly challenging in dynamic scenarios with rapid motion or varying lighting conditions.
Real-Time Video Processing
The ability to process video data in real-time is crucial for immediate decision-making. Delays in processing can lead to compromised safety and functionality.
Low-Power Operation
Many AVs operate on battery power, demanding efficient algorithms and hardware that minimize energy consumption.
These challenges collectively hinder the development of truly intelligent and autonomous systems.
Proposed Solution
A Comprehensive Approach using RZ/V2M and LiveBench
This use case presents a comprehensive solution built around the RZ/V2M evaluation board and LiveBench, a cloud-based testing platform. This potent combination empowers system design engineers to overcome the challenges of Vision AI in AV development.
The RZ/V2M Evaluation Board: A Versatile Development Platform
The RZ/V2M evaluation board serves as the foundation for developing cutting-edge Vision AI applications. This versatile platform offers a suite of features specifically tailored for the needs of AVs:
High-Performance Processor
The RZ/V2M integrates a low-power, yet powerful, MCU capable of executing complex Vision AI algorithms efficiently. This ensures smooth real-time processing without compromising battery life.
Camera Sensor Input Processing
The board features dedicated hardware for pre-processing image data captured by high-resolution cameras. This pre-processing stage optimizes the data for further processing by the AI algorithms.
On-Board Memory and Storage
The RZ/V2M provides ample memory and storage resources to accommodate complex AI models and intermediate processing results.
Development Environment
The board is supported by a comprehensive development environment with software tools and libraries specifically designed for Vision AI applications.
This combination of processing power, dedicated hardware acceleration, and a supportive development environment allows engineers to focus on building innovative Vision AI solutions without getting bogged down in hardware complexities.
Sony IMX568 Sensor: Capturing Clarity in Motion
The RZ/V2M evaluation board can be integrated with the Sony IMX568 image sensor, a powerful addition that tackles the challenge of accurate object detection in dynamic environments. This sensor boasts global shutter technology, a significant advantage over traditional rolling shutter sensors.
Global Shutter Technology
Unlike rolling shutter sensors that capture image data line by line, the global shutter sensor captures the entire image frame simultaneously. This eliminates motion blur and distortion, even under high-speed movement, ensuring crisp and clear images for accurate object detection.
The inclusion of the Sony IMX568 sensor significantly enhances the effectiveness of Vision AI applications in AVs, where precise object identification in motion is paramount.
LiveBench: Optimizing Performance and Validating Application Design Performance
LiveBench plays a crucial role in optimizing and validating the performance of Vision AI applications developed on the RZ/V2M platform. LiveBench offers a comprehensive suite of features specifically designed for Vision AI evaluation:
Remote Testing Environment
Engineers can evaluate the preloaded Vision AI application to test its functionality on the RZ/V2M hardware platform hosted on LiveBench. This eliminates the need for physical access to the hardware, streamlining the evaluation process.
Real-Time Data Visualization
LiveBench provides real-time visualizations of key performance metrics, object detection accuracy, and resource utilization. This allows engineers to identify bottlenecks and optimize their applications for optimal performance.
Comprehensive Test Scenarios
LiveBench integrates an external image rotation system that simulates various motion scenarios. This enables engineers to rigorously test the pr-loaded object detection application under dynamic conditions, replicating real-world situations encountered by AVs.
By leveraging LiveBench’s capabilities, engineers can gain invaluable insights into the performance of the Vision AI applications. This allows for targeted optimization and ensures robust functionality before deployment in real-world environments.
A Step-by-Step Approach to Developing Vision AI Applications
System Design and Algorithm Selection
The initial step involves defining the specific application for your AV. This could involve tasks like object detection and tracking for obstacle avoidance, lane line detection for autonomous navigation, or traffic sign recognition for intelligent traffic management systems. Once the application is defined, engineers can select the appropriate AI algorithms for the task. Popular choices for object detection in AVs include YOLO (You Only Look Once) and SSD (Single Shot MultiBox Detector) due to their efficiency and accuracy.
Model Training and Optimization
The next stage involves training the chosen AI model using a large dataset of labeled images that represent the objects the robot or AV needs to detect. This dataset should encompass various lighting conditions, weather scenarios, and object orientations to ensure the model generalizes well to real-world situations. LiveBench can be a valuable tool in this stage, as it allows engineers to evaluate object detection model on the RZ/V2M hardware platform, providing insights into real-world performance and facilitating model optimization.
Integration and Development
The trained AI model is then integrated into the development environment provided by the RZ/V2M board. Engineers can leverage the available software tools and libraries specifically designed for Vision AI applications to streamline the integration process. This may involve optimizing the model for the specific hardware capabilities of the RZ/V2M MCU to ensure efficient execution.
Testing and Validation with LiveBench
LiveBench becomes a critical tool in this stage. Engineers can evaluate the object detection application by observing the complete setup physically. LiveBench then remotely executes the application on the RZ/V2M hardware, allowing engineers to observe real-time performance metrics like frame rate, object detection accuracy, and resource utilization.
The external image rotation system within LiveBench plays a vital role here. By simulating various motion scenarios through controlled image rotation, engineers can rigorously test their application’s effectiveness in dynamic environments, replicating real-world situations encountered by AVs. This allows for targeted optimization and identifies potential issues with motion blur or object tracking under rapid movement.
Deployment and Real-World Implementation
Following extensive testing and validation on LiveBench, the final Vision AI application is deployed onto the target AV platform. The RZ/V2M evaluation board serves as a powerful development platform, but for real-world deployment, engineers may choose a more robust hardware platform with similar functionalities based on the specific requirements of the AV.
Benefits of the Proposed Solution
The available evaluation setup of RZ/V2M, Sony IMX568 sensor, on LiveBench platform offers several advantages for developing robust Vision AI applications in AVs:
Simplified Development Process
The RZ/V2M platform provides a user-friendly environment with dedicated hardware and software tools, streamlining the development process and minimizing development time.
Enhanced Object Detection Accuracy
The global shutter technology of the Sony IMX568 sensor ensures clear and distortion-free images, leading to improved object detection accuracy, especially in dynamic environments.
Real-Time Performance Optimization
LiveBench’s remote testing capabilities and real-time data visualization empower engineers to optimize their applications for optimal performance, ensuring efficient and smooth operation in real-world scenarios.
Reduced Development Costs
The RZ/V2M evaluation board offers an affordable and accessible platform for prototyping and testing Vision AI applications, minimizing development costs compared to custom hardware development.
Conclusion
The Future of AVs with Vision AI
The proposed solution, leveraging the strengths of RZ/V2M, Sony IMX568 sensor, and LiveBench, empowers system design engineers to develop next-generation Vision AI applications that unlock the full potential of autonomous vehicles. With robust object detection, real-time processing capabilities, and efficient development tools, this solution paves the way for a future where AVs operate with greater intelligence, precision, and safety, revolutionizing industries and shaping the way we interact with the world around us.