This use case explores a reference design for an Automated Urban Traffic Management System using Edge AI. The system tests SK-AM62A-LP evaluation module to enable real-time traffic analysis, aiming to boost efficiency, safety, and precision in monitoring critical intersections. Processing data from multiple cameras concurrently, the design enhances detection accuracy and offers comprehensive coverage. We test Texas Instruments’ SK-AM62A-LP capabilities for multi-camera support, high-quality image processing, and low power consumption. Real-world performance metrics like temperature stability and processing speeds are presented, solidifying the potential of Edge AI in automating urban traffic management.
Improving Traffic Flow and Safety with Automated Intersection Management
Urban traffic congestion poses a significant challenge for modern cities. Traditional management methods struggle to keep pace with the complexities of ever-growing traffic volumes. This use case introduces a reference design for an Automated Urban Traffic Management System that utilizes Edge AI technology. The system focuses on improving real-time monitoring and decision-making at major intersections, a critical aspect of urban traffic flow. By leveraging the processing power of Edge AI at the network’s edge, the design aims to address limitations associated with latency and centralized processing.
Challenges of Urban Traffic Management
Urban traffic management at major intersections poses multifaceted challenges
- Intersection Monitoring: Implement multiple cameras at key intersections to capture real-time footage and employ machine learning algorithms for comprehensive traffic analysis. This enables accurate detection of vehicles, pedestrians, and potential conflicts, facilitating better decision-making for traffic management.
- Congestion Detection: Utilize edge AI capabilities to identify congestion hotspots accurately. Adjust traffic signal timings dynamically and reroute traffic as necessary to alleviate congestion, minimizing delays and improving overall traffic flow.
- Anomaly Detection: Process multiple video streams to detect abnormal events such as accidents or stalled vehicles efficiently. Enhance anomaly detection by cross-referencing information from different camera feeds and automate alerts to emergency services for rapid response.
- Enhanced Accuracy with Multi-Camera Processing: Analyze multiple video streams concurrently to improve accuracy in traffic monitoring. Cross-reference information from different camera feeds to ensure precise detection and enable better-informed decision-making by traffic management authorities.
- Redundancy and Resilience: Offer redundancy in case of camera failures or obstructions by processing multiple video streams. Maintain continuity in traffic monitoring by relying on data from other cameras, enhancing system resilience and ensuring uninterrupted operation.
- Data Insights and Reporting: Generate comprehensive traffic analytics to provide valuable insights for urban planners and transportation agencies. Process data from multiple camera feeds simultaneously to offer rich contextual information about traffic dynamics, aiding in optimizing infrastructure and planning future road developments.
Why SK-AM62A-LP?
An effective solution to these challenges lies in the deployment of an Automatic Traffic Monitoring System utilizing Texas Instruments’ SK-AM62A-LP evaluation module offering advanced capabilities for real-time traffic analysis, safety enhancement, and management on roadways.
Features and Functionality
- Multi-Camera Support: The SK-AM62A-LP supports up to four cameras, providing comprehensive coverage of intersections, highways, and urban roads, crucial for accurate traffic monitoring.
- High-Quality Image Processing: Equipped with an image signal processor supporting up to 5 MP at 60 fps and HDR encoding, the system ensures clear and detailed image capture across various lighting conditions, essential for precise traffic analysis.
- Edge AI Acceleration: The onboard 2 TOPS AI accelerator enables efficient execution of deep learning algorithms directly at the edge, enhancing the system’s ability to perform complex tasks such as object detection, vehicle counting, and classification with minimal latency.
- Real-Time Analytics: Combining powerful AI processing and high-performance cores allows for real-time analysis of traffic patterns, vehicle speeds, lane occupancy, and other pertinent metrics. This enables instant detection of anomalies or congestion, facilitating prompt responses from traffic management authorities.
- Adaptability: The system can dynamically adjust its algorithms based on the specific requirements of different traffic scenarios, ensuring optimal performance and accuracy across various environments, ranging from urban streets to highways.
Can the SK-AM62A-LP live up to these promises?
Validating Performance: Lab Test Results
Let’s uncover the lab test results to see how it performs in real-world conditions.
Power Consumption Analysis
The SK-AM62A demonstrates exceptional power efficiency even for an edge device.
Running a single algorithm, the board consumes approximately 2 watts with a current draw of 2.5 amps.
This value is further reduced for less demanding tasks like image classification.
Power consumption scales with algorithm complexity.
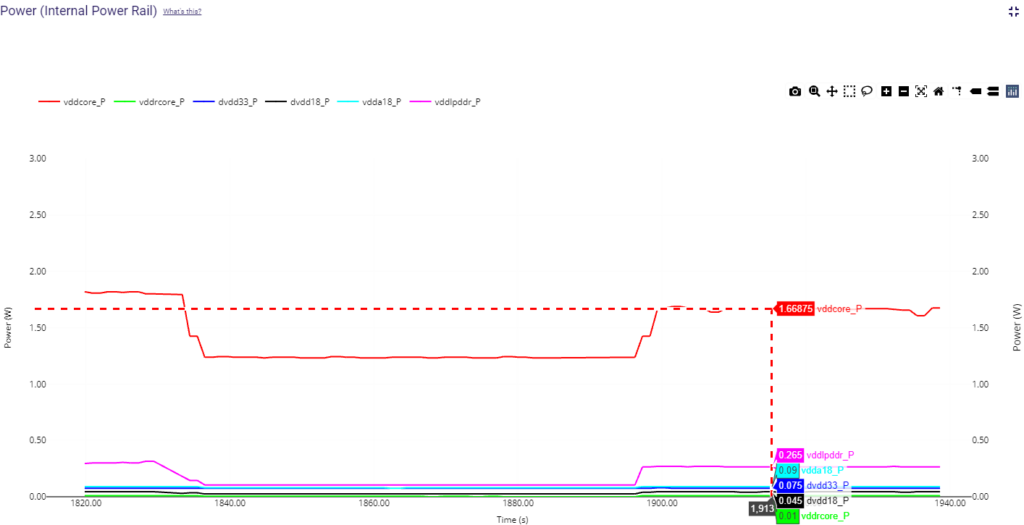
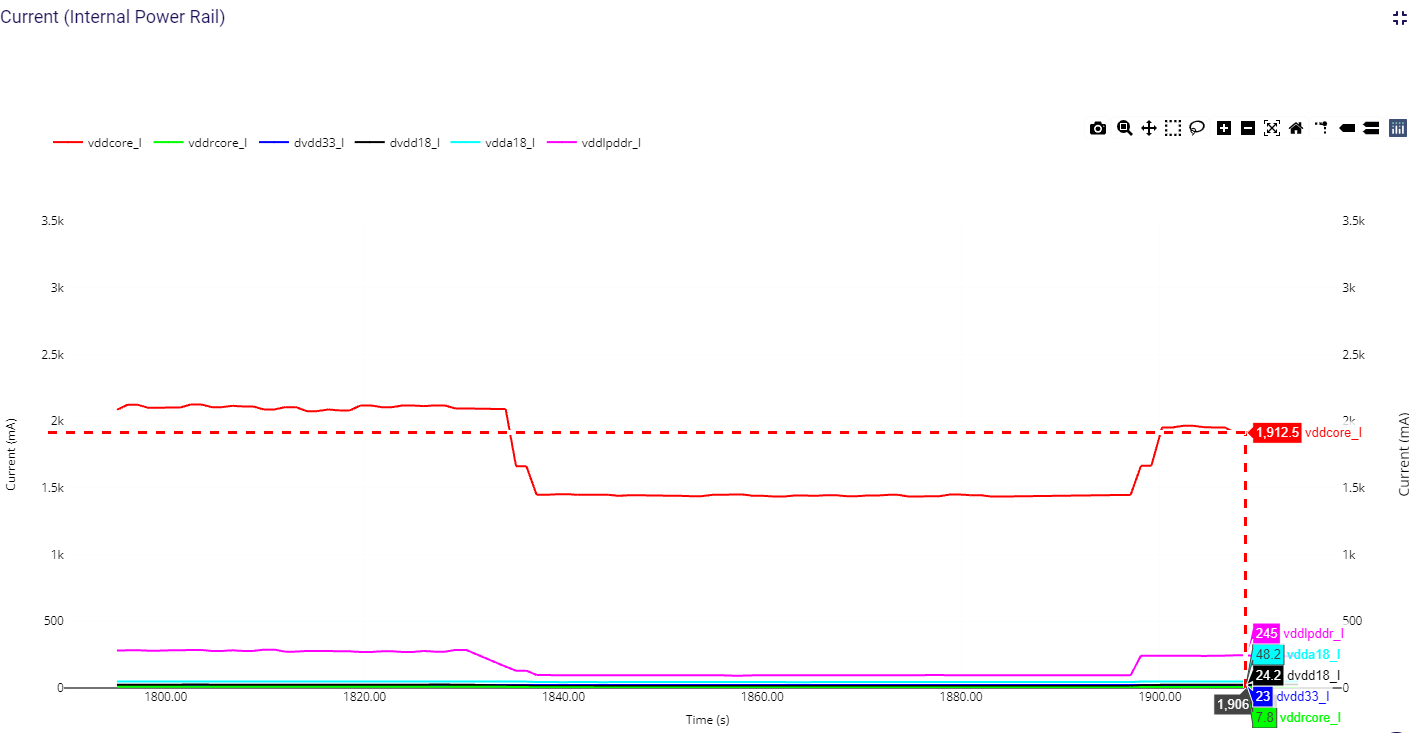
With four camera feeds, running four different algorithms simultaneously, the power consumption is around 2.5 watts while maintaining a total current level below 3 Amps.
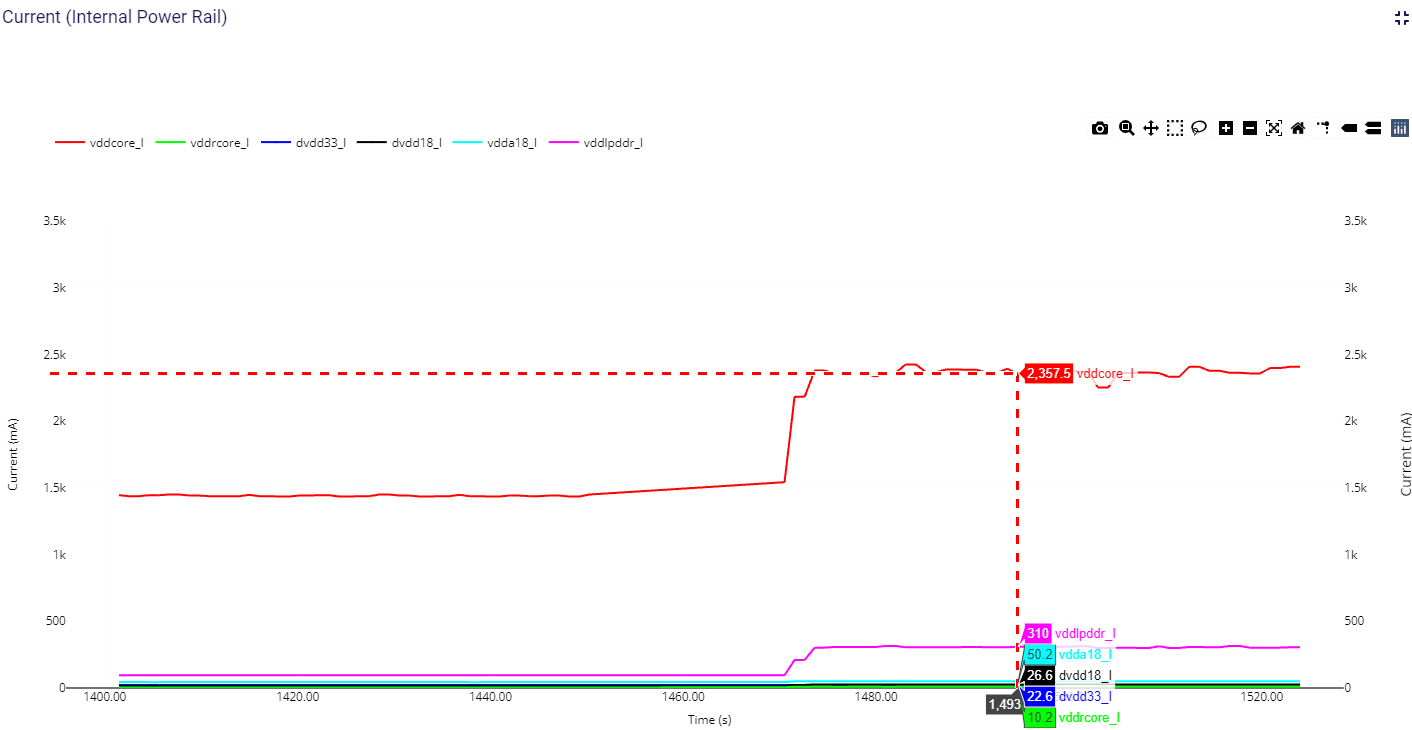
Voltage Regulation
Voltages across various nodes of the power rail are observed to be extremely stable. The power rail structure and voltage levels are shown below:
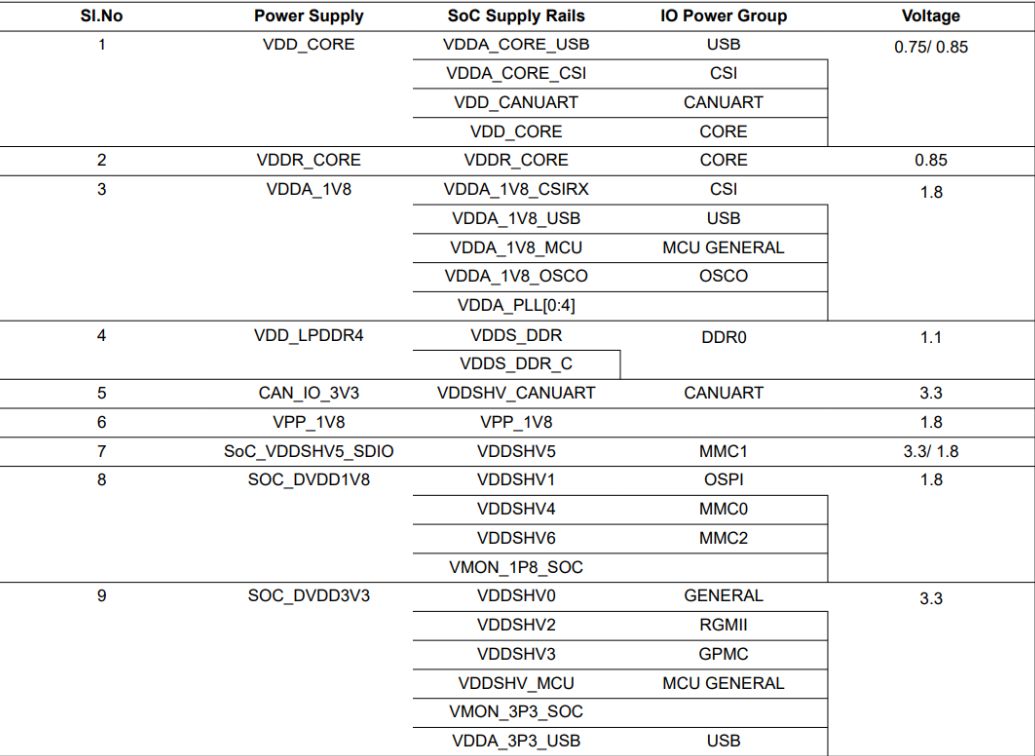
We have measured these voltages experimentally and the result is shown below:
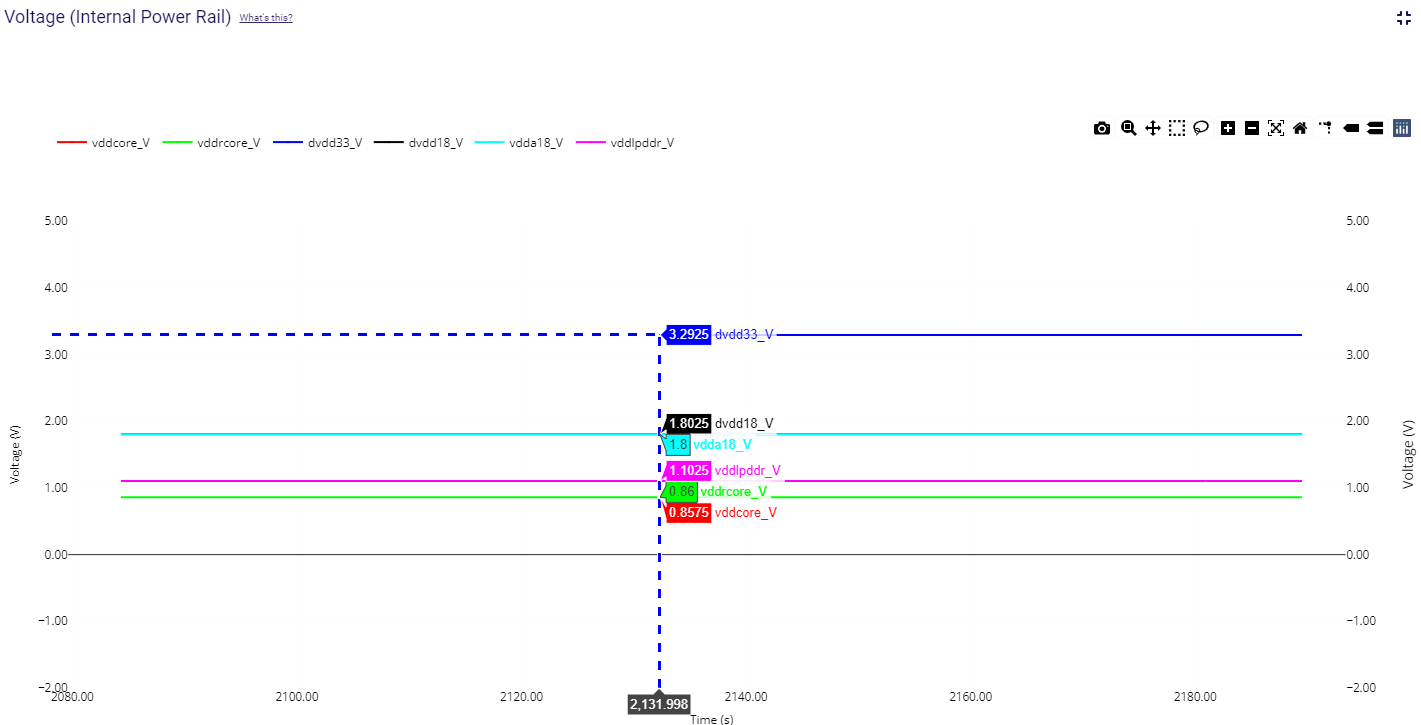
These results are obtained while running four camera feeds that were getting processed simultaneously and one can observe, even in this limiting scenario, the voltage levels are extremely stable maintaining a near zero voltage regulation making it ideal for edge AI applications.
Thermal Performance
Extensive testing has confirmed the board’s excellent thermal management. In a controlled environment, running a single camera feed resulted in a minimal temperature rise of only 2°C.
Processing four feeds simultaneously increased the average temperature by 4-5°C.
With a critical trip temperature of 105°C, the SK-AM62A is well-suited for deployment in harsh outdoor environments.
Model statistics
In case of applications like automatic traffic management, realtime processing and decision making plays a key role.

As observed from the above table, while running an image classification task, the inference time is 7.6 milliSeconds while maintaining a decent frame rate of 30 fps. This type of high speed processing is conducive to real time decision making in critical use cases like traffic management where even a one second delay may cost someone’s life.
These specs vary from algorithm to algorithm and that can be observed from the tables below:



The lowest frame rate has been observed in case of human pose estimation and it is nearly 8.5 frames per second.
While processing all the four camera feeds together, we are getting less than 50 milliseconds of inference time and framerate of nearly 12 frames per second that can be observed from the table below.

Benchmarking Results
To comprehensively evaluate performance, we conducted various benchmarking tests using industry-standard tools like LMBench, Linpack, and Stream. These tests assess various parameters including basic processor operations, floating-point computing performance, and latency. The detailed results are presented below and demonstrate the board’s well-rounded capabilities for real-time edge AI applications.
Linpack:
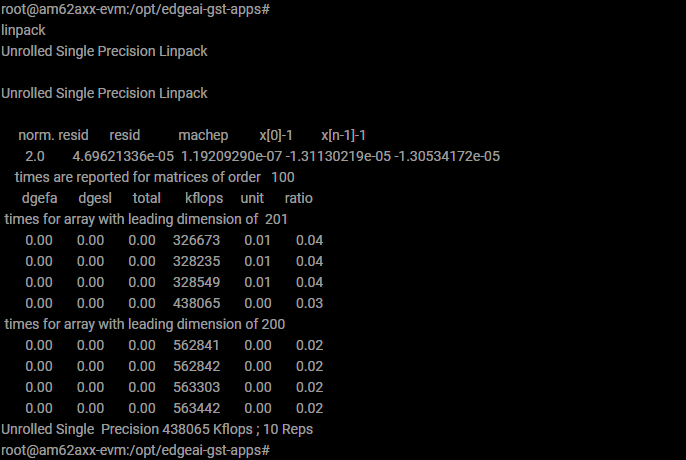
lat_ops:
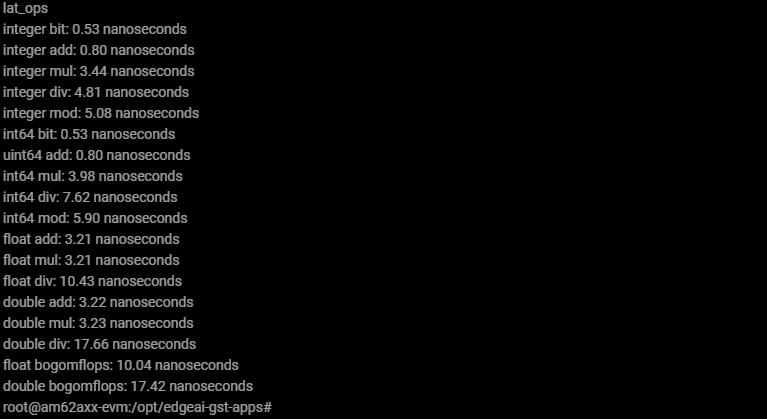
mhz:

stream:
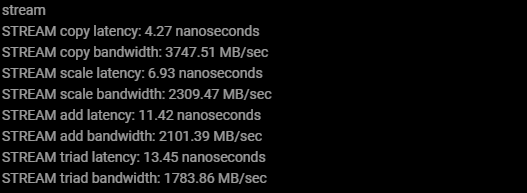
The lab test results showcase impressive results across various parameters. The benchmarking scores demonstrate the board’s all-round capabilities, including basic processor operations, floating-point computing power, and latency. These results solidify the SK-AM62A-LP’s potential as a powerful platform for real-time traffic management applications.
Conclusion
This use case presented a compelling reference design for an Automated Urban Traffic Management System powered by Edge AI and the SK-AM62A-LP evaluation module. The system boasts real-time processing, multi-camera support, and exceptional power efficiency, paving the way for smarter and safer traffic management in urban environments. The successful implementation of such a system hinges on overcoming engineering challenges related to data security, algorithm optimization for edge devices, and ensuring reliable communication between edge and cloud infrastructure.
To empower system design engineers, Tenxer Labs will soon unveil a ready-to-use lab on LiveBench. This lab will allow engineers to validate their designs using the SK-AM62A-LP evaluation module, accelerating development and deployment of automated traffic management solutions.